PhD Thesis
Health-aware Predictive Control Schemes Based on Industrial Processes
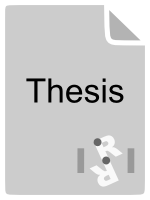
Student/s
Supervisor/s
Information
- Started: 17/04/2015
- Finished: 24/07/2020
Description
This thesis intends to provide theoretical and practical contributions on safety and control of industrial systems, especially in the mathematical form of uncertain systems. The research is motivated by real applications, such as pasteurization plant, water networks and autonomous systems. All of them require a specific control system to provide proper management able to take into account their particular features and operating limits in presence of uncertainties related to their operation and failures from component breakdowns.
Most of the real systems present nonlinear behaviors. One promising approach to deal with non-linear systems is representing them by means of Linear Parameter Varying (LPV) and Takagi-Sugeno (TS) models. In this thesis, an Economic Model Predictive Control (MPC) approach based on LPV/TS models is proposed and the stability of the proposed approach is guaranteed by using a region constraint on the terminal state. Besides, the MPC-LPV strategy is extended to deal with systems with varying delays affecting states and inputs. The control approach allows the controller to accommodate the scheduling parameters and delay change. By computing the prediction of the state variables and delay along a prediction time horizon, the system model can be modified according to the evaluation of the estimated state and delay at each time instant.
To increase the system reliability, anticipate the appearance of faults and reduce the operational costs, actuator health monitoring should be considered. Different strategies are studied for assessing the system health. First, the damage is assessed with the rainflow-counting algorithm that allows estimating the component fatigue and control objective is modified by adding an extra criterion that takes into account the accumulated damage. Besides, two different health-aware economic predictive control strategies that aim to minimize the damage of components are presented.
Then, an economic health-aware MPC approach is developed to determine the components and system reliability in the MPC model using an LPV modeling approach that maximizes the availability of the system by considering system reliability. Then, another approach is proposed using chance-constraint programming to compute an optimal list replenishment policy based on a desired risk acceptability level and aiming to dynamically determine safety stocks in flow-based networks to satisfy non-stationary flow demands.
Finally, an innovative health-aware control approach for autonomous racing vehicles allowing to simultaneously control them at the driving limits and to follow the desired path based on maximization of the battery RUL. The control design is divided into two layers with different time scale, path planner and controller. The proposed approach is formulated as an optimal on-line robust LMI based MPC driven from Lyapunov stability. The controller gain are determined by solving an LPV-LQR problem using LMIs and including an integral action for improving trajectory tracking.
Follow us!